The InstaCities1M Dataset
I’ve have created a dataset of social media images with associated text, InstaCities1M. It’s formed by Instagram images associated associated with one of the 10 most populated English speaking cities all over the world. It has 100K images for each city, which makes a total of 1M images, split in 800K training images, 50K validation images and 150K testing images. The interest of this dataset is: First that is formed by recent social media data. The text associated with the images is the description and the hashtags written by the photo up-loaders. So it’s the kind of free available data that would be very interesting to be able to learn from. Second, each image is associated to one city, so we can use this weakly labeled image classes to evaluate our experiments. All images were resized to 300x300 pixels.
This dataset is published together with the ECCV 2018 MULA Workshop paper “Learning to Learn from Web Data through Deep Semantic Embeddings” [PDF]. A blog post about that paper is available here.
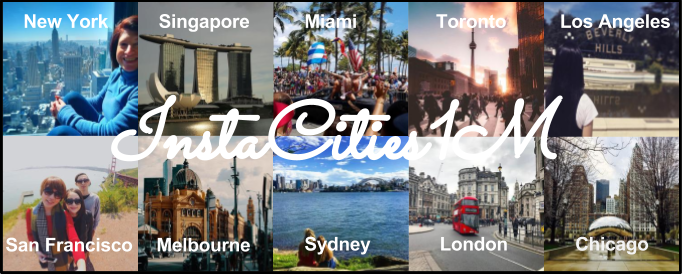
Download InstaCities1M (17.5 GB): CVC (Lab) | Google Drive | Mega
Cities used: London, New York, Sydney, Los Angeles, Chicago, Melbourne, Miami, Toronto, Singapore and San Francisco.
A subset of the dataset, containing only the 100K images with captions associated with New York, is also available.
Download InstaNY100K (1.7 GB): Google Drive
To download the images from Instagram I used InstaLooter, a simple python script that parses the Instagram web without the need API access (The instagram API is only available for approved apps). You can download images quite fast for that, depending on what do you search. I simply did queries with the city names, and I got the 1M images after 2 weeks and some problems.
The code I used to download images from Instagram is available here.
That’s social media data, so it’s very noisy. Instagram does the search by the text associated to the image, and not all images that have the word “london” in the description are from london. Overmore, from the images that were taken in London, only some of them will be representative of the city. We cannot infer that a photo of a dog taken in London inside a house is Londoner (or maybe we can if that’s a typical british race or furniture). ¿What would happen if we used images taken in London GPS coordinates instead of accompanied with the word London? Ok all images would be from London but a lot less representative.
Also, there are some bots and image repetitions (Don’t try this experiment with twitter, bots will ruin them). I did some checks using image size and weight and I found that 98.3% of the images were unique. I removed the repeated ones.
This post describes how i trained a city classifier using InstaCities1M.